Open To Closed AI: Chip Impact
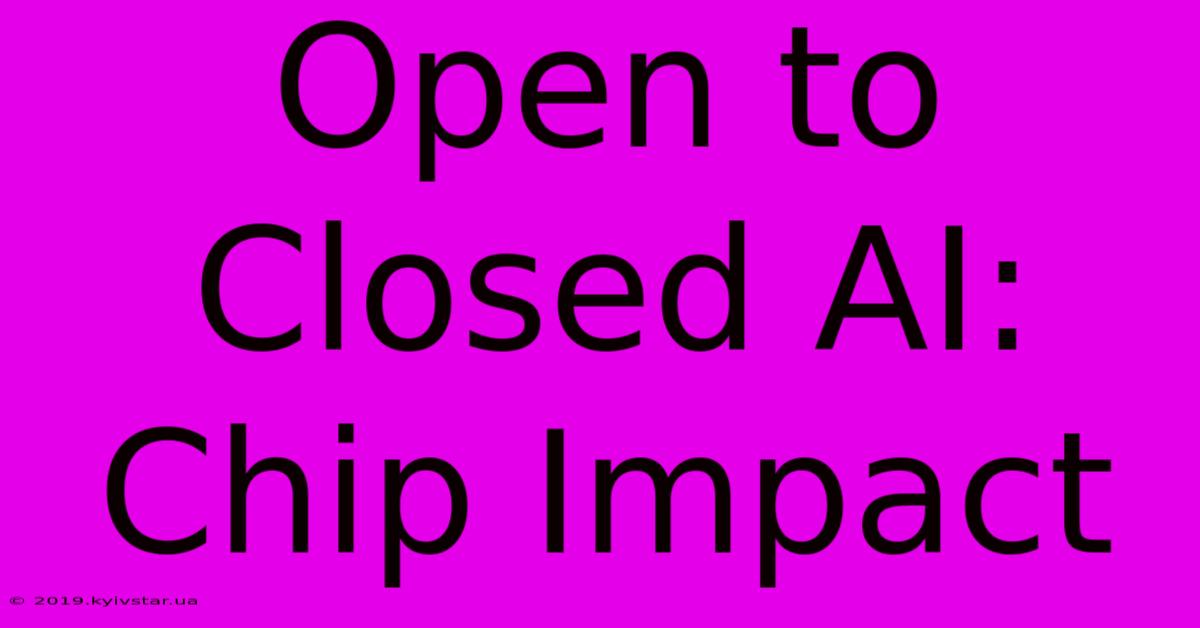
Discover more detailed and exciting information on our website. Click the link below to start your adventure: Visit Best Website. Don't miss out!
Table of Contents
Open to Closed AI: The Profound Impact of Chip Design
The world of artificial intelligence (AI) is rapidly evolving, and at its heart lies the unsung hero: the chip. The shift from open to closed AI architectures is significantly influenced by advancements and limitations in chip design, leading to a complex interplay of benefits and drawbacks. This article will delve into the profound impact of chip design on this transition.
The Open AI Landscape: Collaboration and Customization
Open AI architectures, characterized by their accessible designs and collaborative development, were initially attractive for their flexibility and potential for rapid innovation. This approach fostered a vibrant ecosystem where researchers and developers could freely experiment with algorithms and hardware. However, this openness also presented challenges.
Challenges of Open Architectures:
- Fragmentation: The lack of standardization led to fragmented ecosystems, hindering interoperability and scalability. Different hardware configurations required significant adjustments in software, slowing down progress.
- Security Concerns: Open source designs, while fostering collaboration, also introduced vulnerabilities that could be exploited by malicious actors. Ensuring the security of AI systems built on open architectures is a constant battle.
- Optimization Limitations: While customization was a strength, optimizing performance across diverse hardware platforms was often difficult, leading to suboptimal results compared to tailored solutions.
The Rise of Closed AI: Proprietary Designs and Performance Gains
The move towards closed AI architectures, often driven by major tech companies, prioritizes performance and efficiency. These systems rely on proprietary chip designs optimized for specific AI workloads.
Advantages of Closed Architectures:
- Performance Optimization: Closed systems offer unparalleled performance gains. Chips are meticulously designed to execute specific AI algorithms with maximum efficiency, leading to faster training times and lower energy consumption. Think of the specialized Tensor Processing Units (TPUs) developed by Google, specifically designed for machine learning tasks.
- Enhanced Security: Closed designs allow for better control over security features, reducing vulnerabilities and protecting sensitive data. This is crucial for deploying AI in sensitive applications like healthcare and finance.
- Scalability and Integration: Proprietary chips are designed with scalability in mind, making it easier to deploy large-scale AI systems across multiple devices and data centers. This streamlined integration simplifies deployment and maintenance.
The Chip's Role in the Shift: A Deeper Dive
The shift from open to closed AI is fundamentally driven by the limitations and advancements in chip technology. The demand for faster, more energy-efficient AI processing has pushed the boundaries of chip design, leading to:
Specialized AI Accelerators:
The development of specialized hardware, like GPUs and TPUs, has been a pivotal factor. These accelerators are optimized for matrix operations and other AI-specific tasks, vastly outperforming general-purpose CPUs.
Advanced Memory Architectures:
Efficient memory access is critical for AI performance. High-bandwidth memory (HBM) and other advanced memory architectures play a crucial role in providing the necessary data throughput for demanding AI workloads.
Power Efficiency Improvements:
AI applications often require immense computational power, leading to high energy consumption. Advances in chip design, focusing on power efficiency, are crucial for reducing the environmental impact and cost of running AI systems.
Conclusion: A Balanced Approach?
While the shift towards closed AI architectures offers significant advantages in terms of performance and security, the benefits of open approaches cannot be entirely dismissed. A balanced approach, combining the strengths of both open and closed systems, might be the optimal path forward. This could involve developing open standards for interoperability while still allowing for specialized hardware acceleration within a controlled environment. The future of AI will undoubtedly be shaped by the ongoing evolution of chip design and its impact on the open versus closed debate. The continuing advancements in chip technology will continue to define the trajectory of AI development, presenting both opportunities and challenges along the way.
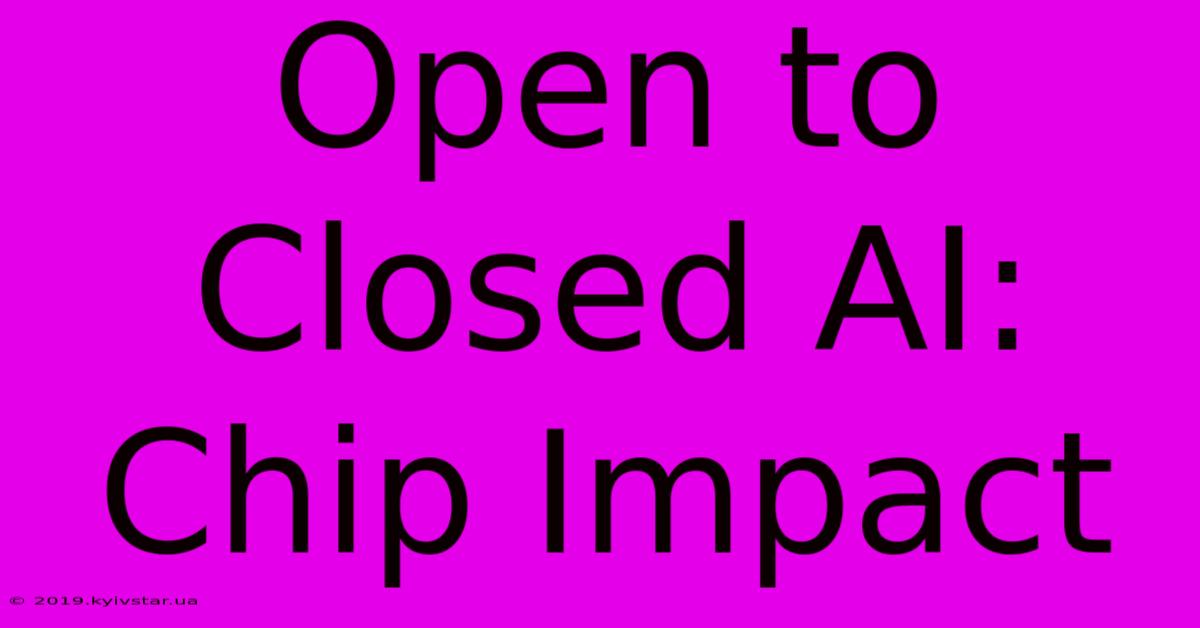
Thank you for visiting our website wich cover about Open To Closed AI: Chip Impact. We hope the information provided has been useful to you. Feel free to contact us if you have any questions or need further assistance. See you next time and dont miss to bookmark.
Featured Posts
-
Russias Ukraine Invasion Current News
Nov 21, 2024
-
Gremio Juventude Donde Y Cuando Ver
Nov 21, 2024
-
Nieuwe Speler Om De Zerbis Keuze
Nov 21, 2024
-
Shin Tae Yong Pimpin Tim Menang Atas Arema
Nov 21, 2024
-
La Anecdota De Nina Pastori En Martinez Y Hermanos
Nov 21, 2024